Four short links: 8 July 2020
AI Weirdness, Experimentation, ML Ops, and Engineer Productivity
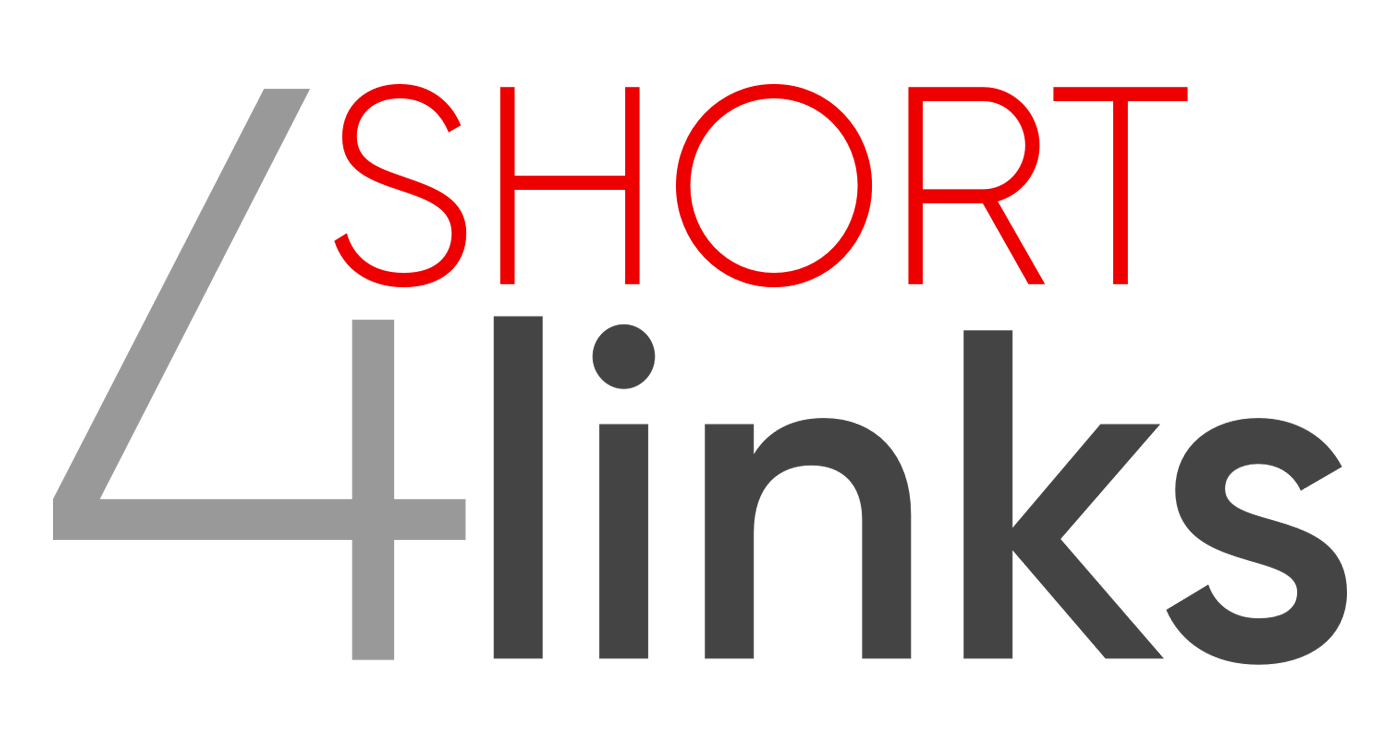
- When Data is Messy — I love stories that illustrate the ways machine learning can draw the wrong conclusions. Researchers at the University of Tuebingen trained a neural net to recognize images, and then had it point out which parts of the images were the most important for its decision. When they asked it to highlight the most important pixels for the category “tench” (a kind of fish) it highlighted human fingers on a green background. Because most of the tench pictures the neural net had seen were of people holding the fish as a trophy. (via Simon Willison)
- Large Scale Experimentation — The internet era has made data-driven decision making easier, faster, and better than ever before. With it come unique challenges and the possibility to rethink how to optimally experiment. We propose a Bayesian setting that implicitly captures the opportunity cost of having multiple interventions to test. Featuring a nifty simulation to help you feel what it is to learn from experiments.
- CI/CD for Machine Learning — an ecosystem of tools. CML helps you bring your favorite DevOps tools to machine learning. Ops for ML projects is interesting because it brings new problems with no widely-known solutions.
- Engineer Productivity — (Charity Majors) Some of the hardest and most impactful engineering work will be all but invisible on any set of individual metrics. You want people to trust that their manager will have their backs and value their contributions appropriately at review time, if they simply act in the team’s best interest. You do not want them to waste time gaming the metrics or courting personal political favor.