Ashish Kakran
More posts from Ashish Kakran
In the early 2000s, most business-critical software was hosted on privately run data centers. But with time, enterprises overcame their skepticism and moved critical applications to the cloud.
DevOps fueled this shift to the cloud, as it gave decision-makers a sense of control over business-critical applications hosted outside their own data centers.
Today, enterprises are in a similar phase of trying out and accepting machine learning (ML) in their production environments, and one of the accelerating factors behind this change is MLOps.
Similar to cloud-native startups, many startups today are ML native and offer differentiated products to their customers. But a vast majority of large and midsize enterprises are either only now just trying out ML applications or just struggling to bring functioning models to production.
Here are some key challenges that MLOps can help with:
It’s hard to get cross-team ML collaboration to work
An ML model may be as simple as one that predicts churn, or as complex as the one determining Uber or Lyft pricing between San Jose and San Francisco. Creating a model and enabling teams to benefit from it is an incredibly complex endeavor.
In addition to requiring a large amount of labeled historic data to train these models, multiple teams need to coordinate to continuously monitor the models for performance degradation.
There are three core roles involved in ML modeling, but each one has different motivations and incentives:
Data engineers: Trained engineers excel at gleaning data from multiple sources, cleaning it and storing it in the right formats so that analysis can be performed. Data engineers play with tools like ETL/ELT, data warehouses and data lakes, and are well versed in handling static and streaming data sets. A high-level data pipeline created by a data engineer might look like this:
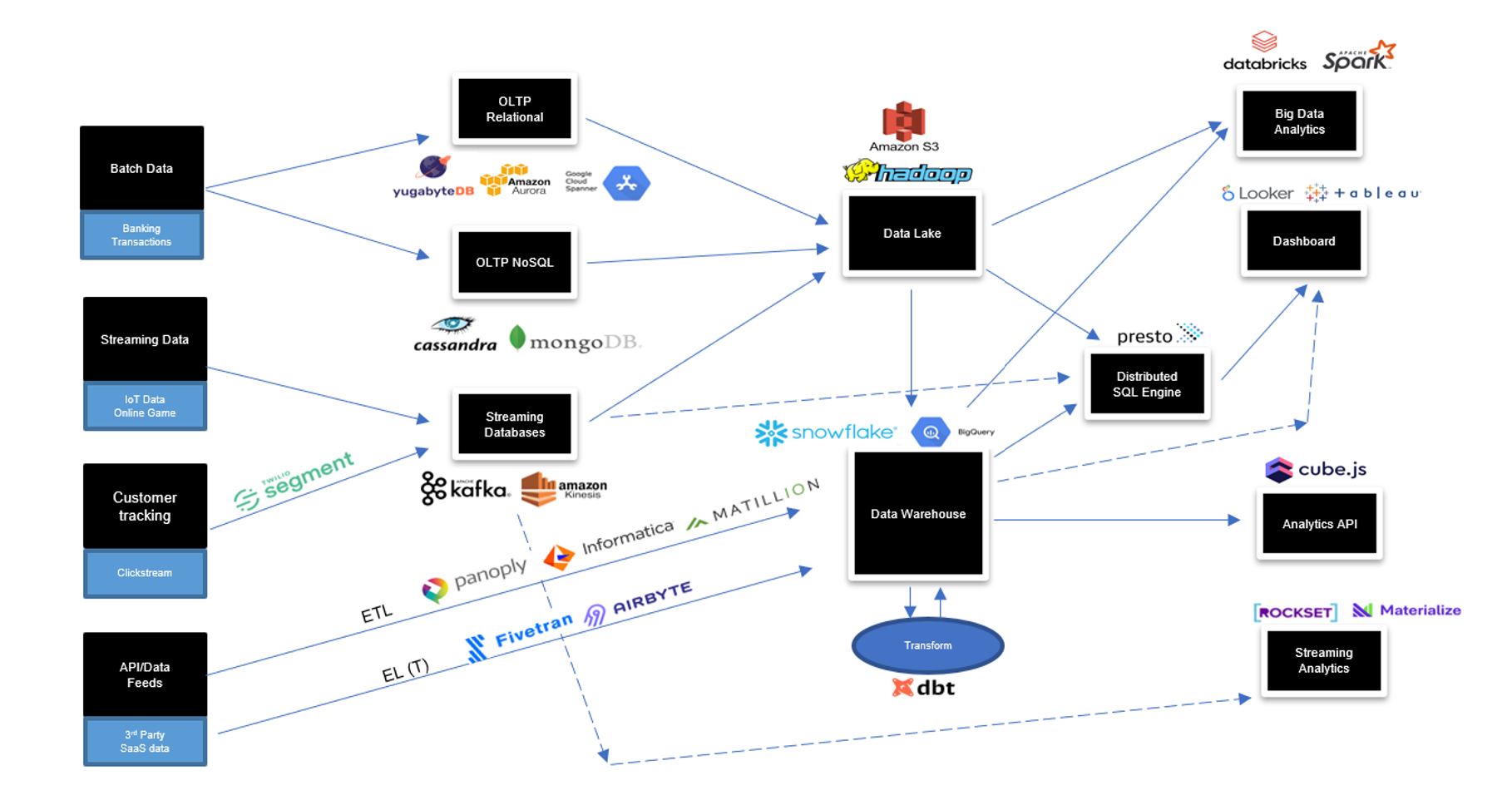
Data scientists: These are the experts who can run complex regressions in their sleep. Using common tools like the Python language, Jupyter Notebooks and Tensorflow, data scientists take the data provided by data engineers and analyze it, which results in a highly accurate model. Data scientists love trying different algorithms and comparing these models for accuracy, but after that someone needs to do the work to bring the models to production.
AI engineers/DevOps engineers: These are specialists who understand infrastructure, can take models to production and if something goes wrong, can quickly detect the issue and kickstart the resolution process.
MLOps enables these three critical personas to continuously collaborate to deliver successful AI implementations.
The proliferation of ML tools
In the new developer-led, bottom-up world, teams can choose from a plethora of tools to solve their problems.
In the diagram below outlining critical steps to do AI correctly, MLOps tools integrate with some or all of the standalone tools that excel at these tasks. Without such tools, it becomes a complex challenge to build, maintain and update ML pipelines that can automatically extract intelligence from vast repositories of data.
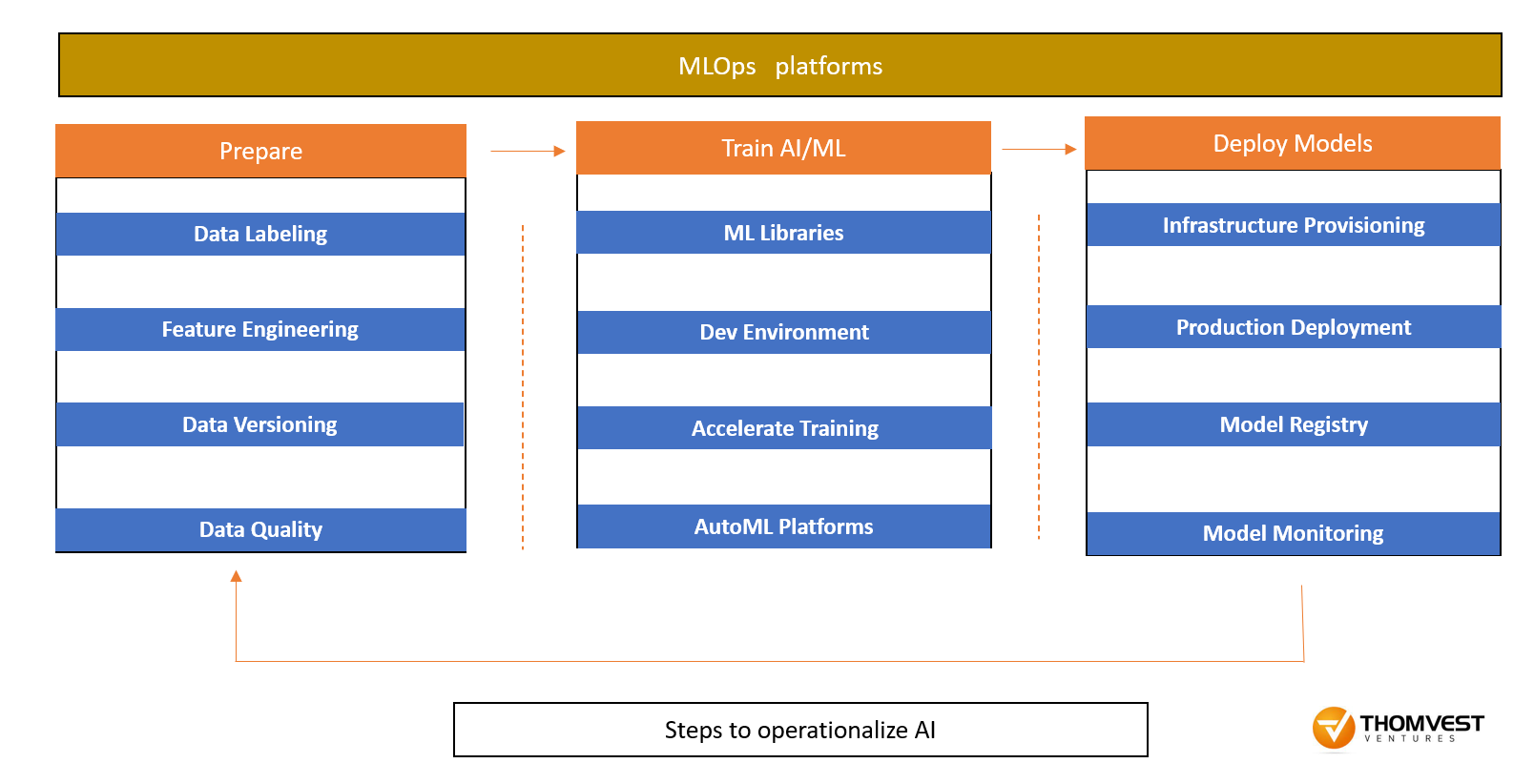
Model lifecycle management is a big pain point
ML models are the core entity that data scientists try to create, optimize, monitor and upgrade. An ML model can be thought of as a black-box software that generates predictions with a high degree of confidence when it is provided with a question and some data. The more accurate the predictions, the more differentiated the experience a company can deliver to its customers.
But unlike software applications, models in production can decay over time, leading to poorer accuracy. Monitoring the performance of models for accuracy, setting fine-tuned alerts and getting the right teams to take corrective action is a tough problem that many MLOps tools are trying to solve today.
The journey from the ML lab to production environments is a hard one
From our conversations with thought leaders in ML infrastructure, we’ve learned that in a large organization, it can take six to nine months for a simple model to move from prototype to production. According to Gartner, only 53% of ML models make it to production today.
MLOps is the missing piece here, and in its absence, simple problems can become a barrier to the successful implementation of ML models. Even a simple question like “What is the definition of a customer?” can be hard to answer precisely. And if this definition changes, ensuring that the update flows through the entire system is a pain point today.
Regulation and compliance
In regulated industries, some parameters just can’t be used for model training. For example, The Federal Reserve Bank’s Regulation B prohibits discrimination against credit applicants on any prohibited basis, such as race, national origin, age, marital status or gender.
Without intelligent alerting and enforcement of policies on model training, organizations may unknowingly violate some industry-specific regulation.
Accelerating adoption of AI in the enterprise
MLOps is similar to DevOps, as it’s also a combination of people, process and technology. The software tools that fall into the MLOps category automate a part of the process required to operationalize AI.
The MLOps space is in its early days today, but it has massive potential because it allows organizations to bring AI to production environments in a fraction of the time it takes today.
What are we excited about?
We are witnessing the data volume explosion in real time — it comes in multiple varieties (structured, unstructured), varying frequency (streaming, real time, static) and large volumes (we’re talking petabytes, not gigabytes anymore). According to Cisco, more network traffic will be created in 2022 than in the first 32 years since the internet started.
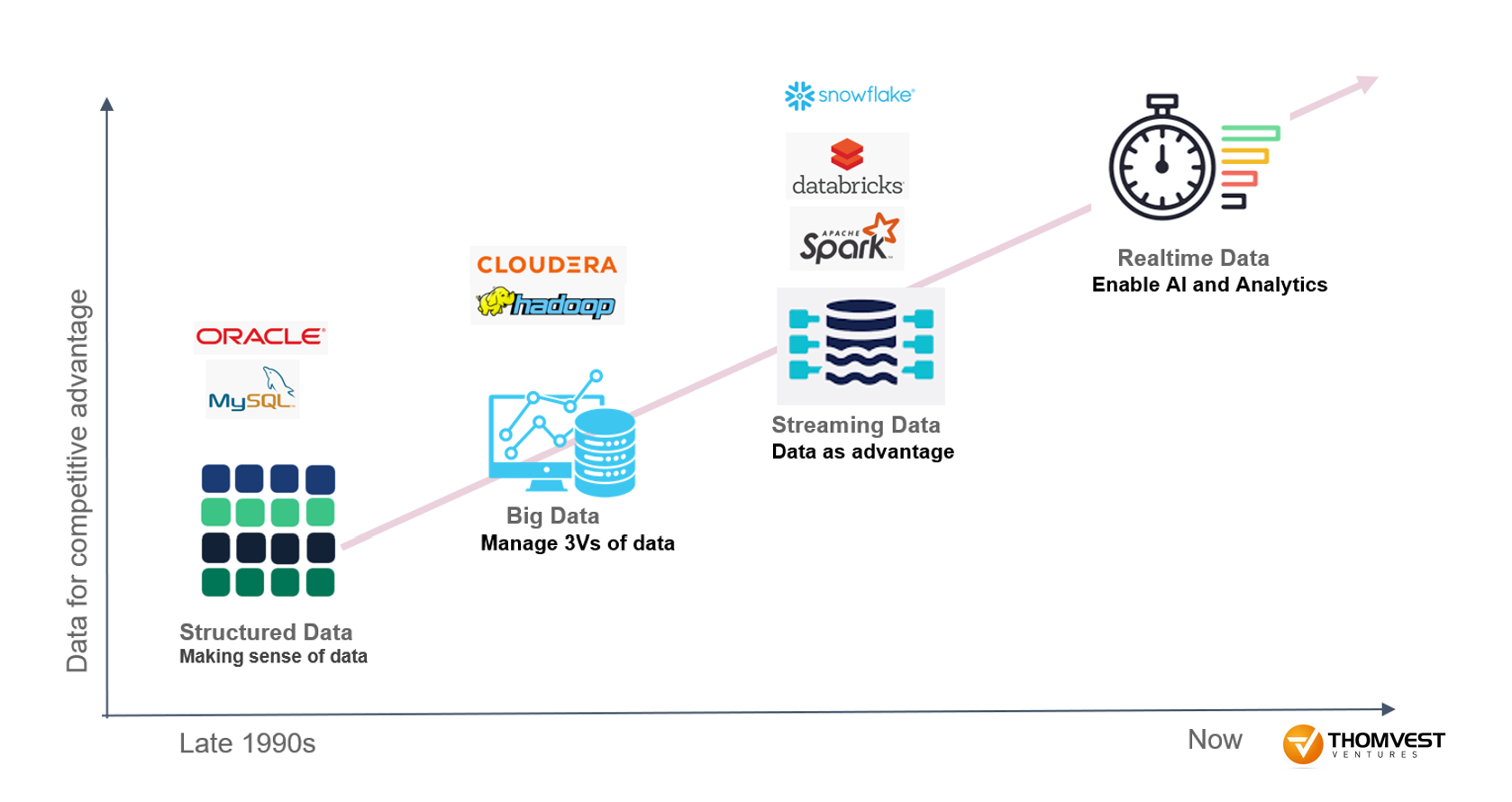
Technology has had to evolve to keep up with the pace of data creation. Each such change in technology creates a massive opportunity for visionary founders to build something interesting. We are excited about the innovation within the data and ML infrastructure space to enable real-time AI and analytics.
Comment