The initial research papers date back to 2018, but for most, the notion of liquid networks (or liquid neural networks) is a new one. It was “Liquid Time-constant Networks,” published at the tail end of 2020, that put the work on other researchers’ radar. In the intervening time, the paper’s authors have presented the work to a wider audience through a series of lectures.
Ramin Hasani’s TEDx talk at MIT is one of the best examples. Hasani is the Principal AI and Machine Learning Scientist at the Vanguard Group and a Research Affiliate at CSAIL MIT, and served as the paper’s lead author.
“These are neural networks that can stay adaptable, even after training,” Hasani says in the video, which appeared online in January. When you train these neural networks, they can still adapt themselves based on the incoming inputs that they receive.”
The “liquid” bit is a reference to the flexibility/adaptability. That’s a big piece of this. Another big difference is size. “Everyone talks about scaling up their network,” Hasani notes. “We want to scale down, to have fewer but richer nodes.” MIT says, for example, that a team was able to drive a car through a combination of a perception module and liquid neural networks comprised of a mere 19 nodes, down from “noisier” networks that can, say, have 100,000.
“A differential equation describes each node of that system,” the school explained last year. “With the closed-form solution, if you replace it inside this network, it would give you the exact behavior, as it’s a good approximation of the actual dynamics of the system. They can thus solve the problem with an even lower number of neurons, which means it would be faster and less computationally expensive.”
The concept first crossed my radar by way of its potential applications in the robotics world. In fact, robotics make a small cameo in that paper when discussing potential real-world use. “Accordingly,” it notes, “a natural application domain would be the control of robots in continuous-time observation and action spaces where causal structures such as LTCs [Liquid Time-Constant Networks] can help improve reasoning.”
Want the top robotics news in your inbox each week? Sign up for Actuator here.
One of the benefits of these systems is that they can be run with less computing power. That means — potentially — using something as simple as a Raspberry Pi to execute complex reasoning, rather than offloading the task to external hardware via the cloud. It’s easy to see how this is an intriguing solution.
Another potential benefit is the black box question. This refers to the fact that — for complex neural networks — researchers don’t entirely understand how the individual neurons combine to create their final output. One is reminded of that famous Arthur C. Clarke quote, “Any sufficiently advanced technology is indistinguishable from magic.” This is an oversimplification, of course, but the point remains: Not all magic is good magic.
The adage “garbage in, garbage out” comes into play here, as well. We all intuitively understand that bad data causes bad outputs. This is one of the places where biases become a factor, impacting the ultimate result. More transparency would play an important role in identifying causality. Opacity is a problem — particularly in those instances where inferred causality could be — quite literally — a matter of life or death.
Paper co-author and head of MIT CSAIL, Daniela Rus, points me in the direction of a fatal Tesla crash in 2016, where the imaging system failed to distinguish an oncoming tractor trailer from the bright sky that backdropped it. Tesla wrote at the time:
Neither Autopilot nor the driver noticed the white side of the tractor trailer against a brightly lit sky, so the brake was not applied. The high ride height of the trailer combined with its positioning across the road and the extremely rare circumstances of the impact caused the Model S to pass under the trailer, with the bottom of the trailer impacting the windshield of the Model S.
Hasani says the fluid systems are “more interpretable,” due, in part, to their smaller size. “Just changing the representation of a neuron,” he adds, “you can really explore some degrees of complexity you couldn’t explore otherwise.
As for the downsides, these systems require “time series” data, unlike other neural networks. That is to say that they don’t currently extract the information they need from static images, instead requiring a dataset that involves sequential data like video.
“The real world is all about sequences,” says Hasani. “Even our perception — you’re not perceiving images, you’re perceiving sequences of images,” he says. “So, time series data actually create our reality.”
I sat down with Rus to discuss the nature of these networks, and help my creative writing-major brain understand about what the emerging technology could mean for robotics, moving forward.
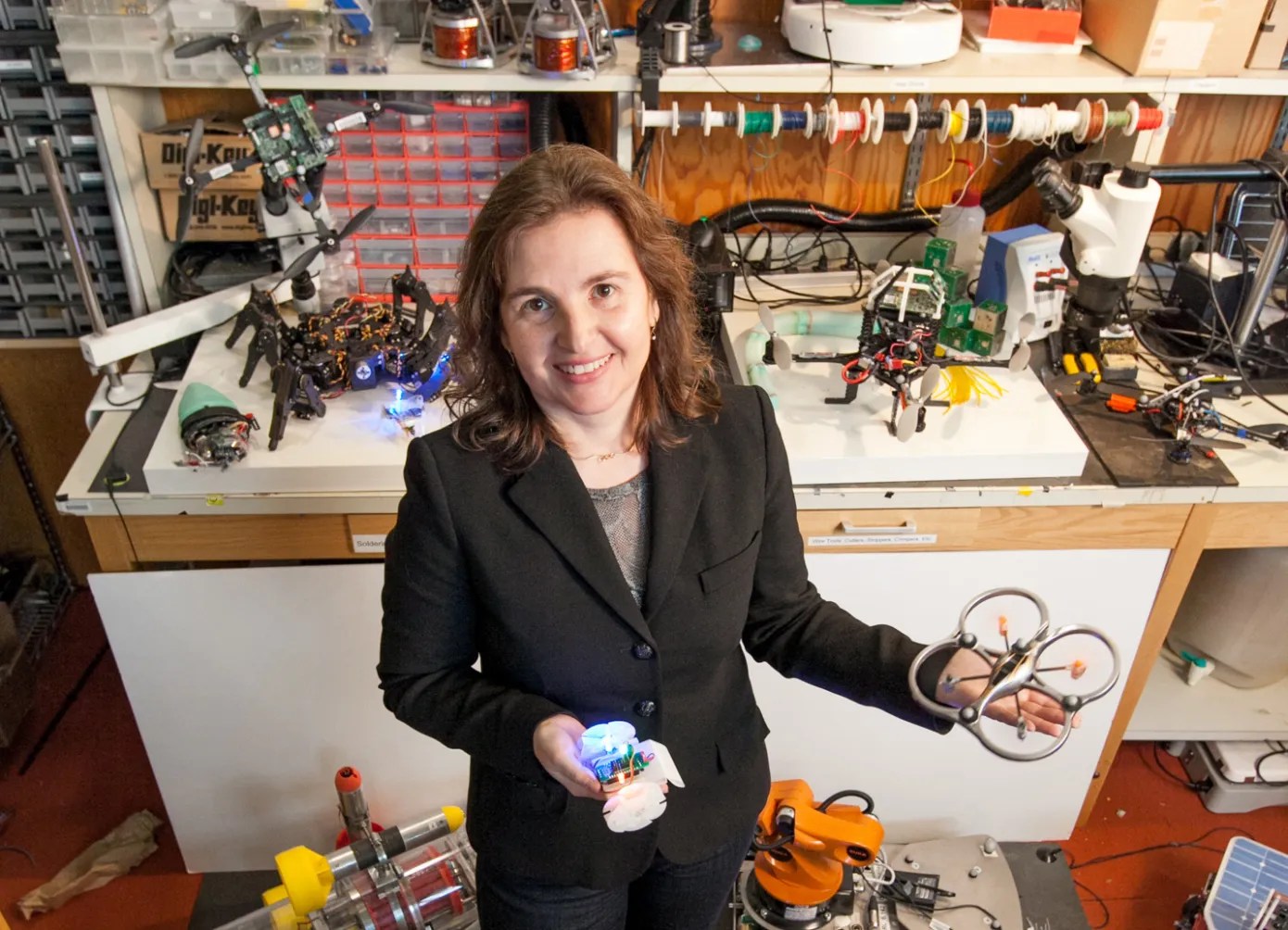
TechCrunch: I get the sense that one of the motivating factors [in the creation of liquid neural networks] are hardware constraints with mobile robotic systems and their inability to run extremely complex computations and rely on these very complex neural networks.
Daniela Rus: That is part of it, but most importantly, the reason we started thinking about liquid networks has to do with some of the limitations of today’s AI systems, which prevent them from being very effective for safety, critical systems and robotics. Most of the robotics applications are safety critical.
I assume most of the people who have been working on neural networks would like to do more with less if that were an option.
Yes, but it’s not just about doing more with less. There are several ways in which liquid networks can help robotic applications. From a computational point of view, the liquid networkers are much smaller. They are compact, and they can run on a Raspberry Pi and EDGE devices. They don’t need the cloud. They can run on the kinds of hardware platforms that we have in robotics.
We get other benefits. They’re related to whether you can understand how the machine learning system makes decisions — or not. You can understand whether the decision is going to meet some safety constraints or not. With a huge machine learning model, it is actually impossible to know how that model makes decisions. The machine learning models that are based on deep networks are really huge and opaque.
And unpredictable.
They are opaque and unpredictable. This presents a challenge, as users are unable to comprehend how decisions are made by these systems. This is really a problem if you want to know if your robot will move correctly — whether the robot will respond correctly to a perceptual input. The first fatal accident for Tesla was due to a machine learning imperfection. It was due to a perception error. The car was riding on the highway, there was a white truck that was crossing and the perceptual system believed that the white truck was a cloud. It was a mistake. It was not the kind of mistake that a human would make. It’s the kind of mistake that a machine would make. In safety critical systems, we cannot afford to have those kinds of mistakes.
Having a more compact system, where we can show that the system doesn’t hallucinate, where we can show that the system is causal and where we can maybe extract a decision tree that presents in understandable human form how the system reaches a conclusion, is an advantage. These are properties that we have for liquid networks. Another thing we can do with liquid networks is we can take a model and wrap it in something we call “BarrierNet.” That means adding another layer to the machine learning engine that incorporates the constraints that you would have in a control barrier function formulation for control theoretic solutions for robots. What we get from BarrierNet is a guarantee that the system will be stable and fall within some bounds of safety.
You’re talking about intentionally adding more constraints.
Yes, and they are the kind of constraints that we see in control theoretic, model predictive control solutions for robots. There is a body of work that is developing something called “control barrier functions.” It’s this idea that you have a function that forces the output of a system within a safe region. You could take a machine learning system, wrap it with BarrierNet, and that filters and ensures that the output will fall within the safety limits of the system. For example, BarrierNet can be used to make sure your robot will not exert a force or a torque outside its range of capability. If you have a robot that aims to apply force that is bigger than what it can do, then the robot will break itself. There are examples of what we can do with BarrieNet and Liquid Networks. Liquid networks are excitig because they address issues that we have with some of today’s systems.
They use less data and less training time. They’re smaller, and they use much less inference time. They are provably causal. They have action that is much more focused on the task than other machine learning approaches. They make decisions by somehow understanding aspects of the task, rather than the context of the task. For some applications, the context is very important, so having a solution that relies on context is important. But for many robotic applications, the context is not what matters. What matters is how you actually do the task. If you’ve seen my video, it shows how a deep network would keep a car on the road by looking at bushes and trees on the side of the road. Whereas the liquid networks have a different way of deciding what to do. In the context of driving, that means looking at the road’s horizon and the side of the road. This is, in some sense, a benefit, because it allows us to capture more about the task.
It’s avoiding distraction. It’s avoiding being focused on every aspect of the environment.
Exactly. It’s much more focused.
Do these systems require different kinds and amounts of training data than more traditional networks?
In our experiments, we have trained all the systems using the same data, because we want to compare them with respect to performance after training them in the same way. There are ways to reduce the data that is needed to train a system, and we don’t know yet whether these data reduction techniques can be applied broadly — in other words, they can benefit all models. Or if they are particularly useful for a model that is formulated in the dynamical systems formulation that we have for liquid networks.
I want to add that liquid neural networks require time series data. A liquid network solution does not work on datasets consisting of static data items. We cannot use ImageNet with liquid neural networks right now. We have some ideas, but as of now, one really needs an application with time series to use liquid networks.
The methods for reducing the amount of information that we feed into our models are somehow related to this notion that there’s a lot of repetition. So not all the data items give you effective new information. There is data that brings and widens information diversity, and then there’s data that’s more of the same. For our solutions, we have found that if we can curate the datasets so that they have diversity and less repetition, then that is the fine way to move forward.
Ramin [Hasani] gave a TEDx talk at MIT. One of the things he said, which makes a lot of sense on the face of it is, “bad data means bad performance.”
Garbage in, garbage out.
Is the risk of bad data heightened or mitigated when you’re dealing with a smaller number of neurons?
I don’t think it’s any different for our model than any other model. We have fewer parameters. The computations in our models are more powerful. There’s task equivalence.
If the network is more transparent, it’s easier to determine where the problems are cropping up.
Of course. And if the network is smaller, it’s easier from the point of view of a human. If the model is small and only has a few neurons, then you can visualize the performance. You can also automatically extract a decision tree for how the model makes decisions.
When you talk about causal relationships, it sounds like one of the problems with these massive neural networks is that they can connect things almost arbitrarily. They’re not necessarily determining causal relationships in each case. They’re taking extra data and making connections that aren’t always there.
The fact is that we really don’t understand. What you say sounds right, intuitively, but no one actually understands how the huge models make decisions. In a convolutional solution to a perception problem, we think that we understand that the earlier layers essentially identify patterns at small scale, and the later layers identify patterns at higher levels of abstraction. If you look at a large language model and you ask, ‘how does it reach decisions?’ You will hear that we don’t really know. We want to understand. We want to study these large models in greater detail and right now this is difficult because the models are behind walls.
Do you see generative AI itself having a major role in robotics and robotics learning, going forward?
Yes, of course. Generative AI is extraordinarily powerful. It really put AI in everyone’s pockets. That’s extraordinary. We didn’t really have a technology like this before now. Language has become a kind of operating system, a programming language. You can specify what you want to do in natural language. You don’t need to be a rock-star computer scientist to synthesize a program. But you also have to take this with a grain of salt, because the results that are focused on code generation show that it’s mostly the boiler plate code that can be generated automatically by large language models. Still, you can have a co-pilot that can help you code, catch mistakes, basically the co-pilot helps programmers be more productive.
So, how does it help in robotics? We have been looking at using generative AI to solve complex robotics problems that are difficult to solve with our existing methods […] With generative AI you can get much faster solutions and much more fluid and human-like solutions for control than we get with model predictive solutions. I think that’s very powerful. The robots of the future will move less mechanistically. They’ll be more fluid, more humanlike in their motions. We’ve also used generative AI for design. Here we need more than pattern generation for robots. The machines have to make sense in the context of physics and the physical world.
Comment